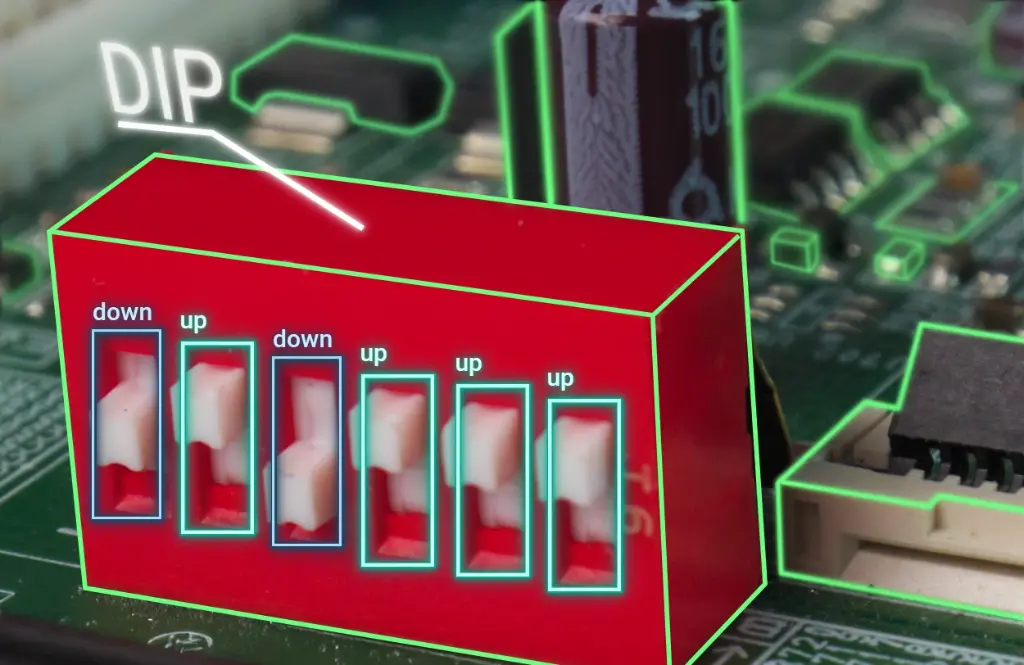
From human to AI:
Quality inspection 4.0
From the basics to the latest achievements in quality testing
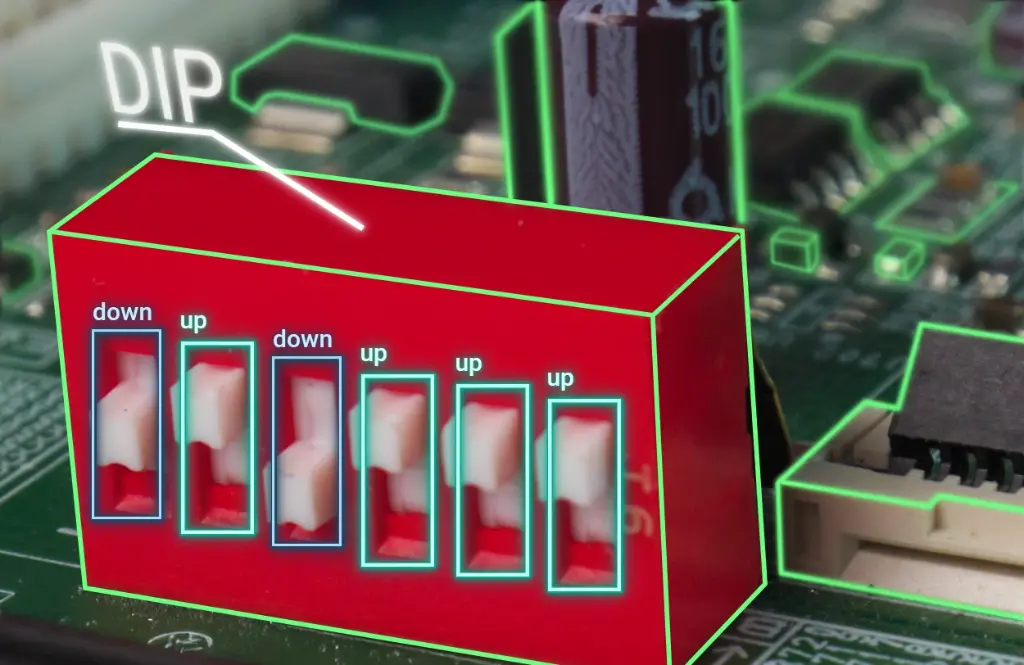
What is camera-based worker guidance?
We explain briefly and concisely how worker guidance works and what it is good for
Quality controls in various forms have been around for as long as products and assemblies have been manufactured. More recently, however, new challenges have arisen that have called for adequate solutions. Due to increasingly complex products, a growing number of product variants and increasing production volumes, quality control has now become a significant cost factor.
The answer to the new challenges is flexible automated quality controls. Instead of relying solely on the human eye, cameras are used to check the quality of parts and assembly processes on the basis of images. This is an innovation with huge potential and one that has not yet been fully developed. But before we get to the latest developments, let's first remind ourselves of the basic principle of camera-based quality control.
How does image-based quality control work?
The basis of image-based quality control is the comparison between an actual image and a target image. The target image is a photo that is taken of a correct component or construction step before the actual quality inspection. It serves as a standard against which the success of a production step is measured. During the actual quality inspection, the actual image is generated and compared with the target image. If the deviation between these two images is too great, the component is found to be faulty and rejected. A sufficient match leads to a positive result and the component is classified as defect-free.
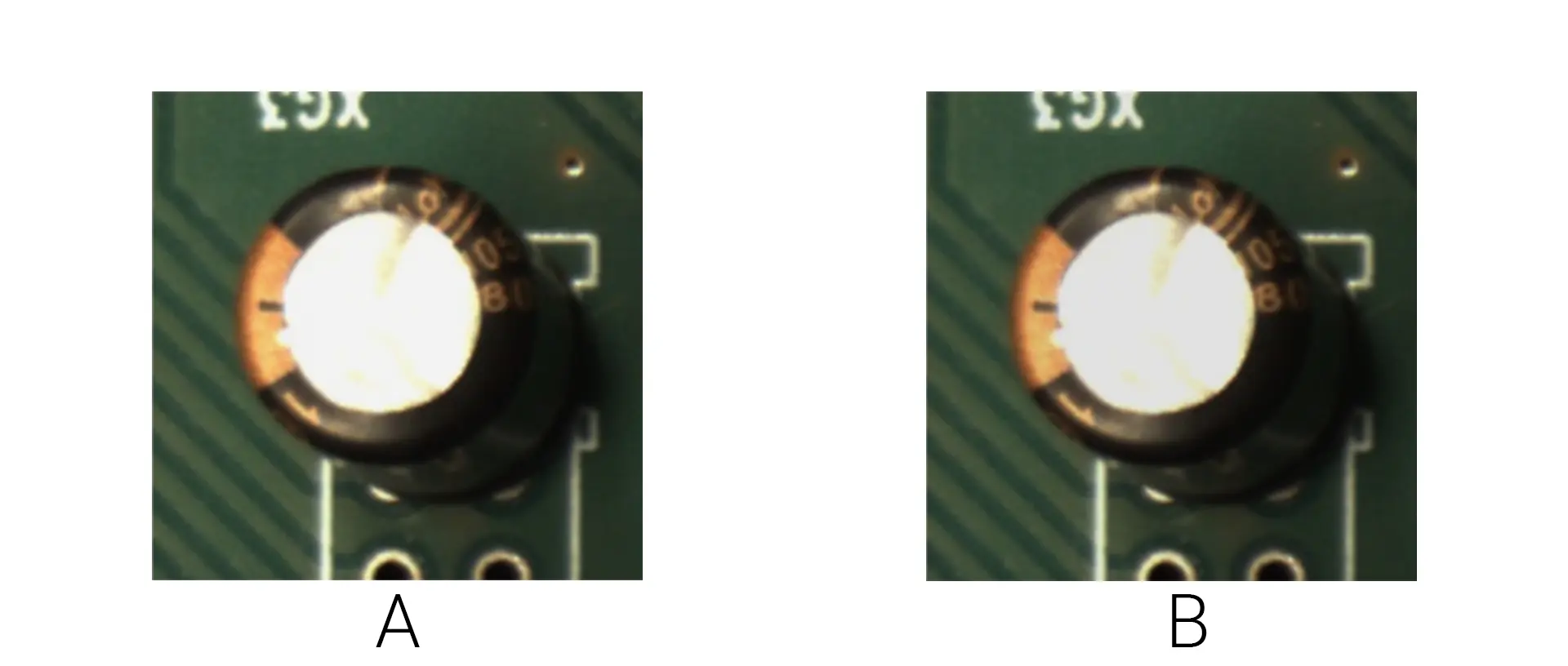
Picture B was made a little darker afterwards to simulate a slightly different lighting situation.
As simple as this may sound at first, there are a few pitfalls that come with quality control. If you were to simply compare two images pixel by pixel, you would get a constant stream of pseudo errors (errors are displayed even though they don't actually exist). Let's take a look at an example. If you compare image A and image B, everyone would probably agree that they are sufficiently identical and that the two capacitors shown must surely be the same component.
However, if you look at two identical positions, you get two clearly different color values.
Even if they are barely visible to the naked eye, the individual pixels have different values
Image B is actually slightly darker than image A. In reality, such differences are usually much greater. If you think of a hall with sunlight, for example, the lighting situation would change significantly depending on whether the sun is shining or not. Humans can make this transfer without any problems and realize that it is still the same component. For a computer, on the other hand, these are simply different values at first glance and therefore not the same component.
So there is much more to quality inspections. Let's take a closer look at this below.
Algorithms and artificial intelligence in quality control
A distinction must first be made between image processing and computer vision. In image processing, images are broken down into their individual pixels, which are then analyzed and, if necessary, modified using algorithms. One example of this is edge detection. Neighboring pixels of the image are examined. If there are sudden differences in brightness or color between two neighboring pixels, one pixel is assigned the value 1 (= white) and the other the value 255 (= black). Neighboring pixels that are similar to one of these two pixels are assigned the same value. This creates a black and white image in which the edges of objects - i.e. where neighboring pixels are most different - are highlighted.
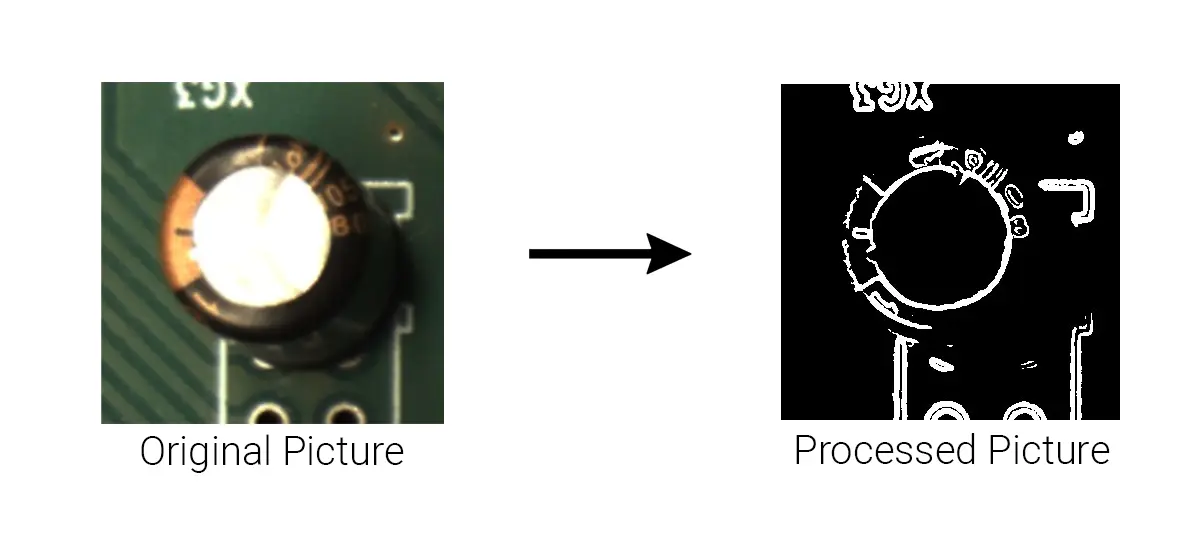
Images processed in this way offer several advantages for quality control. If an unprocessed target and actual image were to be compared instead, pseudo errors would occur much more frequently. Even the tiniest deviations in the recording conditions would cause pixel values (i.e. color and brightness) to deviate between the comparison images and the comparison would produce a negative result, even though the component is actually OK.
Even if image processing makes quality controls significantly more robust against environmental influences, a limit is quickly reached if deviations are too large. This is where computer vision comes into play. Through the use of artificial intelligence, systems are equipped with the ability to understand the content of an image, i.e. to “see” what is being depicted. This ability is based on previously trained AI models. To create these, a large number of images with corresponding labels are required.
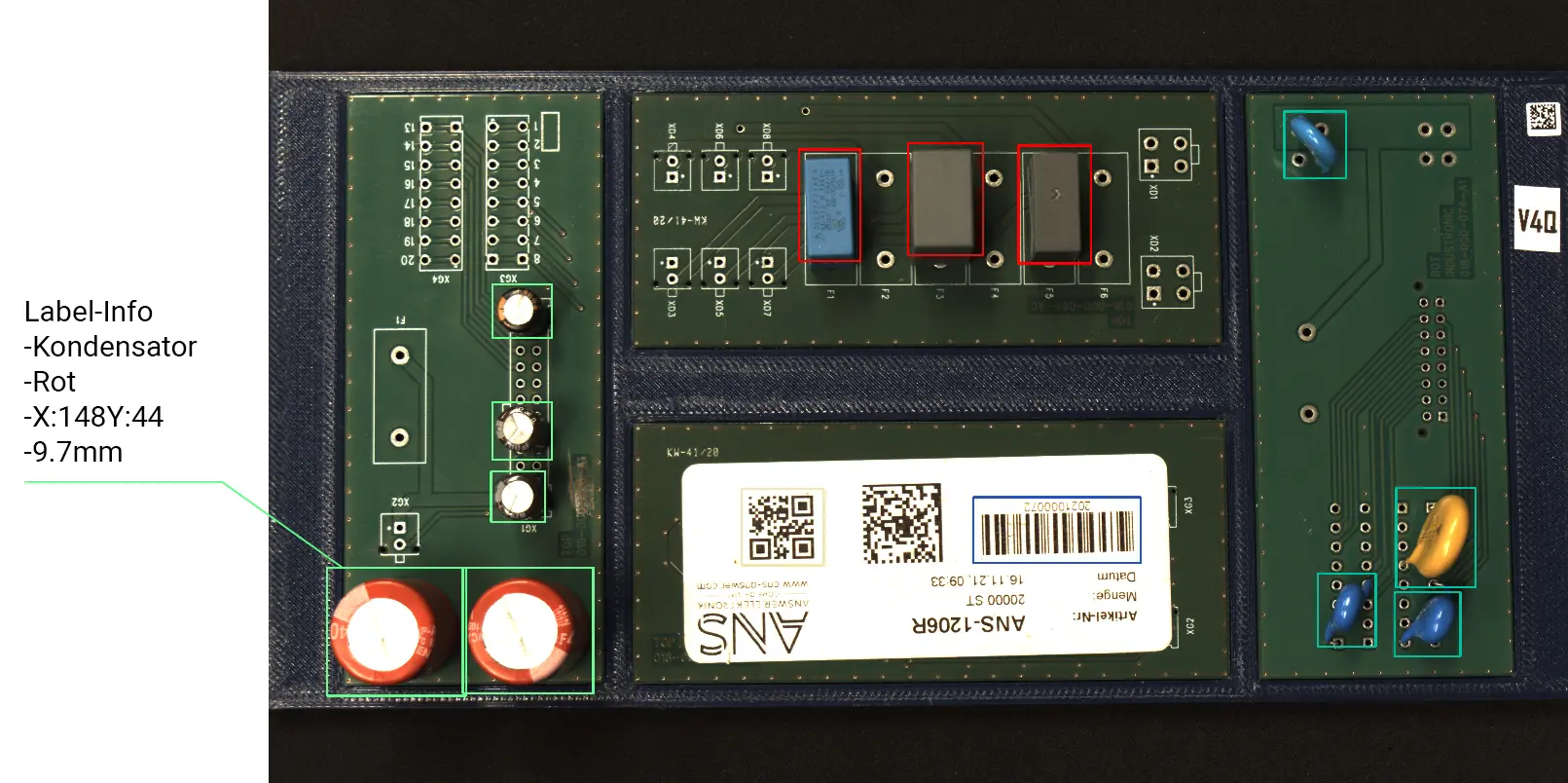
During labeling, components are marked and provided with information such as the name of the component, color, size, position, etc.. Based on this detailed information, the AI learns to link image elements with the corresponding information. You can think of it like a small child. It may see a chair every day, but will only be able to say on its own that it is a chair when it receives the information “this is a chair”.
Use of AI in image-based quality control
In contrast to the conventional quality inspection explained at the beginning, AI does not just rely on the comparison between the actual and target image. Instead, the program “understands” what can be seen on the image, can recognize objects and determine how high the probability is that this is the correct component.
We have already noted in the previous chapters that conventional quality inspections have problems with variable conditions. A changing light situation or position of the component in relation to the camera can make the inspection difficult or even impossible - even with processed images. The advantage of an AI model is that it does not have to be limited to a single target image. It has previously been fed with a wealth of different images of the same component and has built up a deeper understanding of what characteristics make up this component.
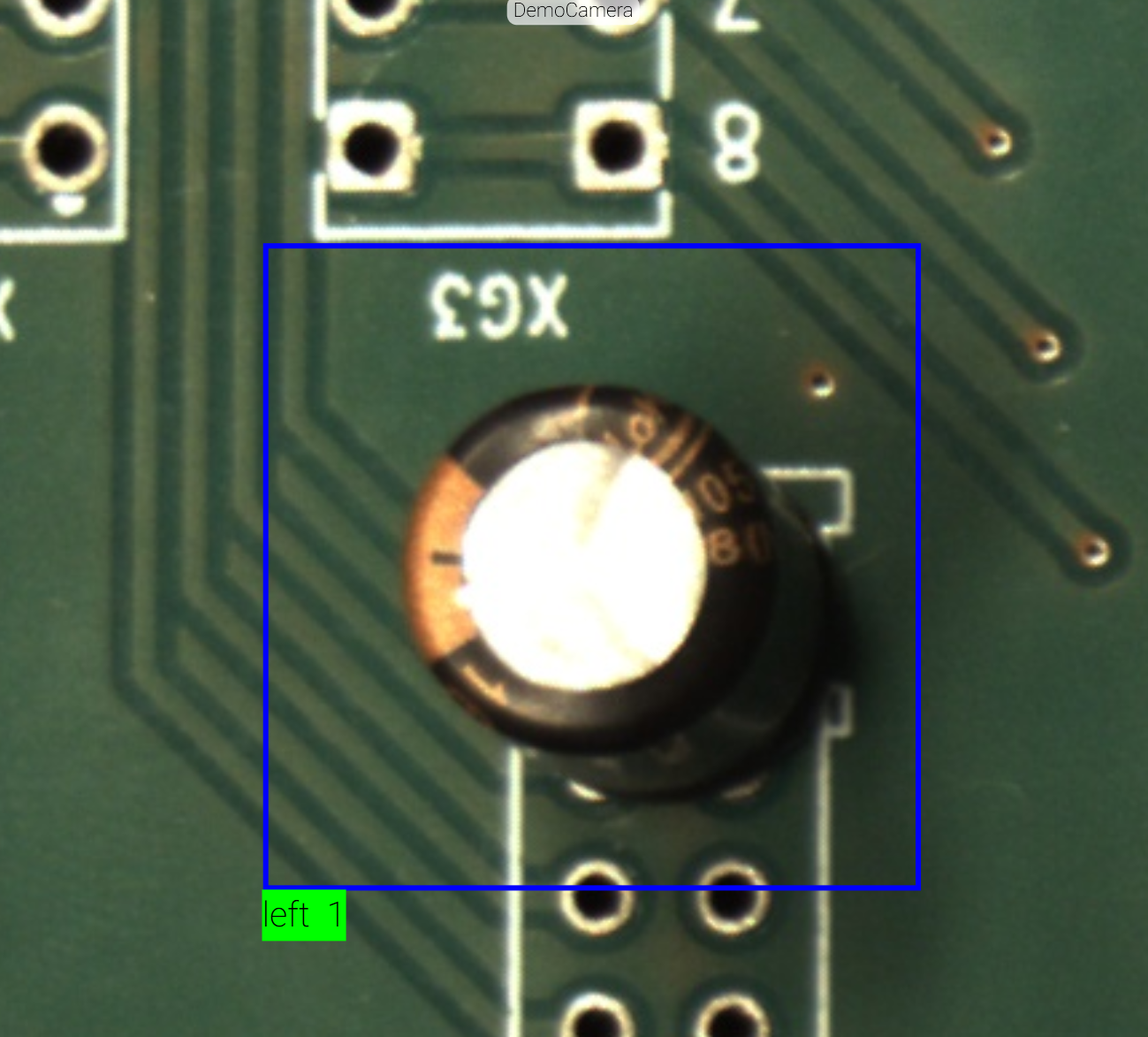
The AI not only recognizes the component as a capacitor, but also in which direction it is polarized
This means that components can be correctly identified even in different positions and in different variants. In addition, more detailed properties of individual parts can also be checked. For example, with the appropriate training, the system not only knows that a capacitor can be seen in the image, but also that the polarity is aligned to the left.
From AOI to worker guidance: Quality inspection 4.0
Quality inspections are required in various industries and for different areas of application. From electronics and automotive to medical technology and aerospace, each industry has its own unique challenges that an inspection system must be able to meet. This diversity results in the enormous potential that AI integration holds in quality control.
With ModOFFICE, Vision4Quality has developed software that harnesses precisely this potential for companies. With just a few clicks, AI models can be created based on your own data. These can be downloaded directly and used for both worker guidance and AOI (Automated Optical Inspection).
With ModPCB, Vision4Quality offers a matching AI-capable worker guidance system that also offers many other features to increase the quality and efficiency of assemblies. The flexible ModSPOT AOI system also works on the basis of artificial intelligence and benefits from the advantages associated with this level of innovation.
Conclusion
By using AI, the number of pseudo errors and false positives is reduced to a minimum, which drastically increases the efficiency of quality inspections. At the same time, machine vision opens up new possibilities for checking components and work steps that would otherwise have to be carried out manually. The disadvantages of AI, such as high computing power requirements, are becoming less and less important, especially with regard to ever better and affordable hardware.
The use of artificial intelligence in quality control is therefore undoubtedly a forward-looking technology that companies should definitely keep in mind when choosing their inspection systems.
Author: Frederick Warken
More information about ModPCB Back to the blog